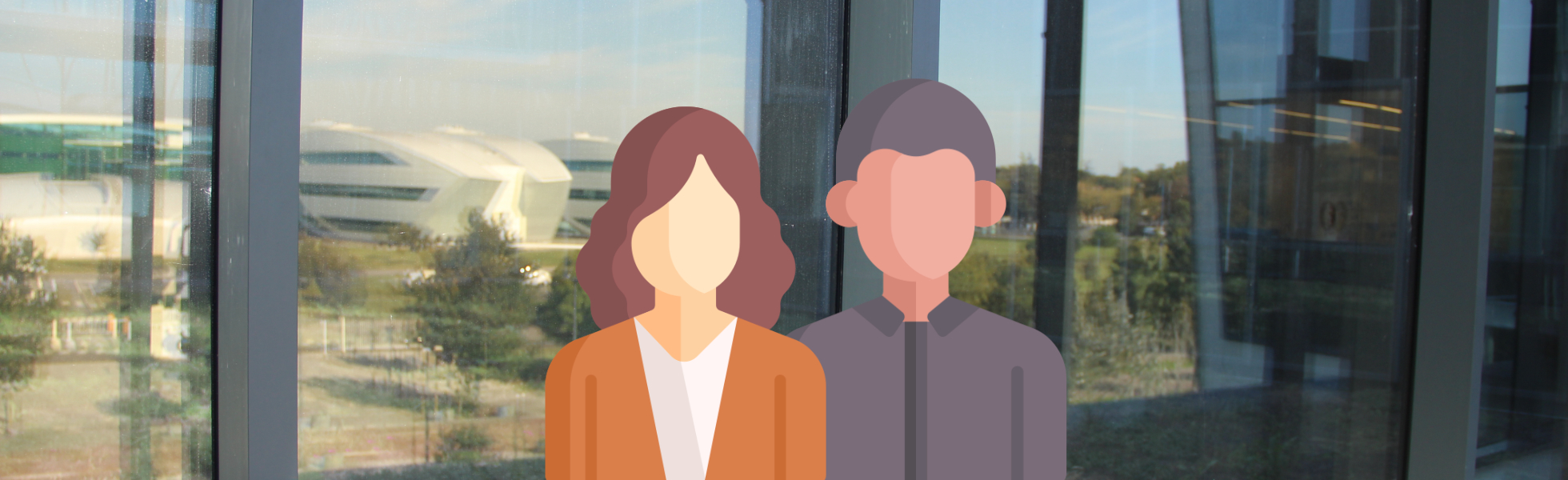
HERVE AVET-LOISEAU & JILL CORRE
Molecular alterations and heterogeneity
within myeloma cells.
Our objective, mainly translational, is to improve the therapeutic management of patients with multiple myeloma (MM), a cancer of the bone marrow, and to prolong their survival. To do this, we have created a national network which has enabled us to constitute the largest bio-bank of tumors in the world, bringing together the clinical and evolutionary characteristics of thousands of patients.
Personalized treatment according to chromosomal changes.
In the recent years, many novel drugs were registered making the therapeutic choice complex for physicians. Our goal is to guide them in choosing the right combination of drugs for the right patients. More specifically, we want to improve the identification of high-risk patients in this era of new drugs, because the most efficient strategies will mostly benefit to newly diagnosed high-risk patients. Recently, we described a 6-markers based score to accurately and routinely stratify MM patients (Perrot et al, JCO 2019). We are massively sequencing these tumors in order to identify subgroups of patients most likely to respond to specific treatments, in order to help clinicians in their choices. We have recently shown 60% of the early relapse patients have no known prognostic genomic alterations. Our project aims to identify, by high-throughput sequencing, the new genomic anomalies that predict myeloma patient’s clinical outcome.
Subclonal evolutions in myeloma
The identification of MM subclones, has considerably changed our view of the mechanisms underlying MM resistance. Still, mainly for technical limitations, MM clonal heterogeneity has been poorly defined. We recently acquired a 10xGenomics Chromium platform, allowing transcriptomic, CNV, and ATACSeq analysis at the single cell (sc) level. Using this “multi-omic” approach, our objectives are to describe the subclonal landscape of each patient at the time of diagnosis and their first relapse, and to analyze the residual tumor cells at the time of response to treatment in order to determine which subclones have been selected. By integrating variable unicellular omics data (RNA, CNV, ATAC, mutations), we will be able to understand the molecular determinants of this selection. The data obtained could have a major impact on our understanding of subclonal selection with a given treatment (treatment-induced selection or natural history), and whether the early-selected subclones will be the main one at the time of relapse.
Figure 1: Single cell RNA sequencing identifies different subclones of MM at diagnosis and relapse. Representative t-SNE plot of CD138+ myeloma cells at diagnosis. Targeted sequencing of a patient’s tumour cells at diagnosis (outer circle) and relapse (inner circle).
Figure 2: Overall survival of patients according to time to first relapse (late in red, early in blue) and known cytogenetic risk factors (low risk continuous line, high risk discontinuous line).